老师,帮忙看下这个问题?
来源:6-25 TensorFlow-ssd 模型测试
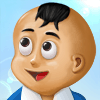
baby猫
2019-08-26
代码如下:
import numpy as np
import sys
import tensorflow as tf
import glob
import cv2
from object_detection.utils import ops as utils_ops
This is needed since the notebook is stored in the object_detection folder.
sys.path.append("…")
What model to download.
Path to frozen detection graph. This is the actual model that is used for the object detection.
PATH_TO_FROZEN_GRAPH = “/home/babycat/mooc/datas/widerface/model/resnet50-v1-fpn/pb/frozen_inference_graph.pb”
List of the strings that is used to add correct label for each box.
PATH_TO_LABELS = “/home/babycat/mooc/py3_tensorflow/models/research/object_detection/data/face_label_map.pbtxt”
detection_graph = tf.Graph()
with detection_graph.as_default():
od_graph_def = tf.GraphDef()
with tf.gfile.GFile(PATH_TO_FROZEN_GRAPH, ‘rb’) as fid:
serialized_graph = fid.read()
od_graph_def.ParseFromString(serialized_graph)
tf.import_graph_def(od_graph_def, name=’’)
im_path_list = glob.glob("/home/babycat/mooc/datas/widerface/test-image/*")
Size, in inches, of the output images.
IMAGE_SIZE = (256, 256)
def run_inference_for_single_image(image, graph):
with graph.as_default():
with tf.Session() as sess:
# Get handles to input and output tensors
ops = tf.get_default_graph().get_operations()
all_tensor_names = {output.name for op in ops for output in op.outputs}
tensor_dict = {}
for key in [‘num_detections’, ‘detection_boxes’, ‘detection_scores’,
‘detection_classes’, ‘detection_masks’]:
tensor_name = key + ':0’
if tensor_name in all_tensor_names:
tensor_dict[key] = tf.get_default_graph().get_tensor_by_name(tensor_name)
if 'detection_masks' in tensor_dict:
# The following processing is only for single image
detection_boxes = tf.squeeze(tensor_dict['detection_boxes'], [0])
detection_masks = tf.squeeze(tensor_dict['detection_masks'], [0])
# Reframe is required to translate mask from box coordinates to image coordinates and fit the image size.
real_num_detection = tf.cast(tensor_dict['num_detections'][0], tf.int32)
detection_boxes = tf.slice(detection_boxes, [0, 0], [real_num_detection, -1])
detection_masks = tf.slice(detection_masks, [0, 0, 0], [real_num_detection, -1, -1])
detection_masks_reframed = utils_ops.reframe_box_masks_to_image_masks(
detection_masks, detection_boxes, image.shape[1], image.shape[2])
detection_masks_reframed = tf.cast(
tf.greater(detection_masks_reframed, 0.5), tf.uint8)
# Follow the convention by adding back the batch dimension
tensor_dict['detection_masks'] = tf.expand_dims(detection_masks_reframed, 0)
image_tensor = tf.get_default_graph().get_tensor_by_name('image_tensor:0')
# Run inference
output_dict = sess.run(tensor_dict, feed_dict={image_tensor: image})
# all outputs are float32 numpy arrays, so convert types as appropriate
output_dict['num_detections'] = int(output_dict['num_detections'][0])
output_dict['detection_classes'] = \
output_dict['detection_classes'][0].astype(np.int64)
output_dict['detection_boxes'] = output_dict['detection_boxes'][0]
output_dict['detection_scores'] = output_dict['detection_scores'][0]
if 'detection_masks' in output_dict:
output_dict['detection_masks'] = output_dict['detection_masks'][0]
return output_dict
for image_path in im_path_list:
im_data = cv2.imread(image_path)
sp = im_data.shape
im_data = cv2.resize(im_data, IMAGE_SIZE)
output_dict = run_inference_for_single_image(im_data, detection_graph)
for i in range(len(output_dict[‘detection_scores’])):
if output_dict[‘detection_scores’][i] > 0.6:
bbox = output_dict[‘detection_boxes’][i]
y1 = int(IMAGE_SIZE[0] * bbox[0])
x1 = int(IMAGE_SIZE[1] * bbox[1])
y2 = int(IMAGE_SIZE[0] * bbox[2])
x2 = int(IMAGE_SIZE[1] * bbox[3])
cv2.rectangle(im_data, (x1, y1), (x2, y2), (0, 255, 0), 2)
cv2.imshow("im", im_data)
cv2.waitKey(0)
1回答
-
会写代码的好厨师
2019-08-28
纬度没对上,给图片数据➕一个纬度变成1✖️256✖️256✖️3应该就好了
012019-09-10
相似问题