pass
来源:4-7 生成tfrecords文件
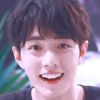
战战的坚果
2020-02-21
for x_batch, y_batch in dataset.take(steps_per_shard):
for x_example, y_example in zip(x_batch, y_batch):#解batch
老师,此时的数据集是之前的8个属性与类标签结合起来,相当于一行9个属性的形式,现在从数据集中取出前steps_per_shard个batch,我想请教的是:获取到steps_per_shard个batch后,是如何赋值给 x_batch, y_batch 的,又是怎样实现对batch解绑的,赋值给 x_example, y_example的,解绑是指将9个属性拆分成8+1吗?
写回答
1回答
-
正十七
2020-02-24
如下面的代码所示:
def parse_csv_line(line, n_fields = 9): defs = [tf.constant(np.nan)] * n_fields parsed_fields = tf.io.decode_csv(line, record_defaults=defs) x = tf.stack(parsed_fields[0:-1]) y = tf.stack(parsed_fields[-1:]) return x, y def csv_reader_dataset(filenames, n_readers=5, batch_size=32, n_parse_threads=5, shuffle_buffer_size=10000): dataset = tf.data.Dataset.list_files(filenames) dataset = dataset.repeat() dataset = dataset.interleave( lambda filename: tf.data.TextLineDataset(filename).skip(1), cycle_length = n_readers ) dataset.shuffle(shuffle_buffer_size) dataset = dataset.map(parse_csv_line, num_parallel_calls=n_parse_threads) dataset = dataset.batch(batch_size) return dataset
在csv_reader_dataset中,我们在map函数中对每一行进行解析。
在parse_csv_line中,我们把每一行给拆成了前八个和后一个,即x和y。这样csv_reader_dataset返回的dataset里,每一个batch都是两个元素,即x_batch和y_batch。
而batch解绑定直接用了for循环,如下,并不是9拆8+1。
with tf.io.TFRecordWriter(filename_fullpath, options) as writer: for x_batch, y_batch in dataset.skip(shard_id * steps_per_shard).take(steps_per_shard): for x_example, y_example in zip(x_batch, y_batch): writer.write(serialize_example(x_example, y_example)) all_filenames.append(filename_fullpath)
012020-02-28